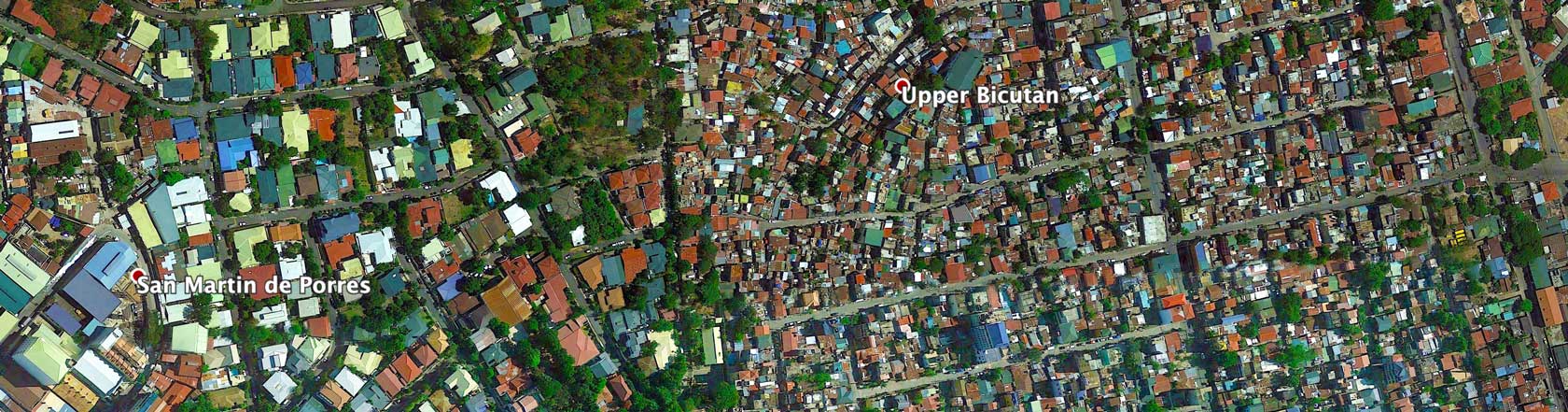
Zero in on the Philippines’ most vulnerable communities – with the click of a mouse
In barangay Sapang Uwak, an Aeta community not too far from Mount Pinatubo in Porac, Pampanga, most households lack access to running water, sanitary toilets, or electricity. In fact, the community’s some 1,200 residents are estimated to be among the poorest 20% in the Philippines.
But you might not be able to tell this by looking at provincial poverty data from the Philippine government. In 2015, the Philippine Poverty Census ranked Pampanga as the province with the 4th lowest poverty incidence in the country. And while the census does indicate that 3.22% of the families in Pampanga are poor, it can’t tell you where exactly in the province these poor families live.
If you’re a development organization that needs up-to-date, accurate, and detailed socio-economic data to make sure your programs target the most vulnerable communities, you might be familiar with this dilemma.
Government surveys, while authoritative, are often only done every three to five years at the regional or provincial level. Without more granular or recent data to guide their policies and programs, development organizations and government agencies risk investing their limited resources in the wrong priorities and places.
Luckily, a solution is within reach.
At Thinking Machines, we use machine learning and high-resolution satellite imagery to reliably estimate wealth in areas small as 18 square kilometers. With the click of a mouse and for a fraction of the cost of running on-the-ground surveys, you can have up-to-date estimates of wealth and poverty for an entire province, or even the entire country, in near real-time.
Using open source deep learning libraries such as Google’s Tensorflow and Pytorch, we developed models that can automatically extract features from satellite images that are correlated with wealth, such as buildings and roads. Our deep learning models also are trained using powerful machines hosted on Google Compute Engine, with Google Cloud Storage (GCS) as our data lake.
Interested in learning more? Learn more about our methodology by reading our technical note or reach out to us for a demo: